Scraping LinkedIn Data
10x Faster
With AI
Scraping LinkedIn Data
10x Faster With AI
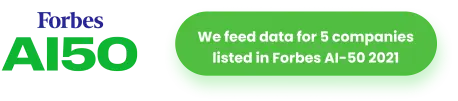
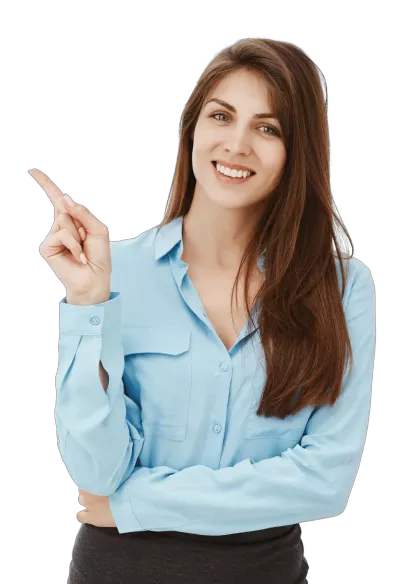
AI-Driven Data Extraction
Real-time Data Updates
Seamless Data Integration
High-Level Accuracy
Anti-Blocking Mechanisms
Customizable Extraction Rules
NO SET-UP COST
NO INFRA COST
NO CODING
4X
Rapid increase of your wealth
30%
Decrease your expenses wisely
1M+
Trusted regular active users
USED BY
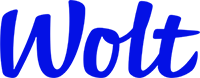
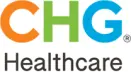


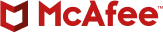
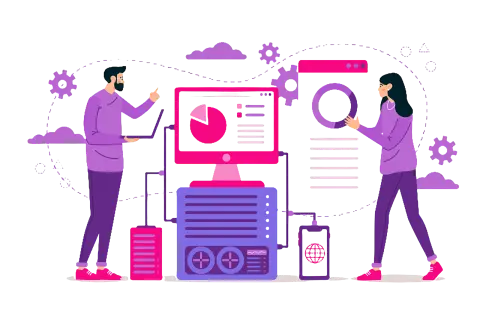
Get Profile Information of LinkedIn Users Easily by Scraping LinkedIn Data with Our AI-Powered LinkedIn Scraping Tool
LinkedIn dominates professional networking, attracting millions of professionals. Demand for innovative tools to access insights from profiles is increasing, leading to a growing need for scraping LinkedIn data.
AI technology can unlock LinkedIn user profiles’ vast information, revolutionizing data-driven decision-making. Our LinkedIn data scraping tool is designed to transform how professionals collect and utilize this valuable professional data.
Outsource Bigdata combines Artificial Intelligence (AI) technology with web scraping capabilities to extract useful data and insights from LinkedIn is known as an AI-augmented LinkedIn scraper. LinkedIn is a professional networking site that has a large database of user profiles, company information, job advertisements, and other resources. The AI-augmented scraper navigates LinkedIn’s complex web architecture intelligently, allowing for rapid and precise data extraction.
Scrape all public LinkedIn data with LinkedIn scraping tool. Our LinkedIn web scraping services include extracting profiles, ID, position, timestamp, URL, city, country code, region, description, education, experience, avatar, about, languages, posts, people also viewed, groups, courses, certifications, recommendations, jobs, and more. All this is extracted and parsed from publicly available products and category information.
What is Meant by Scraping LinkedIn Data?
LinkedIn web scraping is the process of obtaining data or information from LinkedIn’s website or database using automated tools or software. Profile information such as name, job title, company, location, education, and skills may be extracted.
Scraping LinkedIn data helps in lead generation, recruitment, market research, and competitive analysis. LinkedIn, on the other hand, prohibits scraping its website without authorization, and doing so might result in account suspension or legal ramifications.
Types of Data Obtained by Scraping LinkedIn
LinkedIn is a great source of professional information that may be scraped for a variety of purposes. The following are some of the most popular data taken from LinkedIn:

Profile Information
It includes name, job title, employer, location, education, abilities, and other professional details.
Company Information
This covers the company’s name, size, industry, location, and other pertinent information.
Articles or Posts
It collects the most recent posts, articles, and liked content from your new prospects, important clients, or future recruitment.
Contact Information
Email addresses, phone numbers, and social media profiles, among other contact details that individuals choose to make public, are examples of contact information.
Likes or Comments Data
This data is useful since it lists the people who are aware of the topic, contribute to the debate, and are engaged in their professional lives. Such information is useful for generating leads.
Job Postings
This covers job names, job descriptions, prerequisites, and other information relevant to LinkedIn job listings.
Group Information
This includes the name of the group, its members, discussions, and other information about LinkedIn groups.
Auto-Connect Action
Based on your profile choices, the scraper automatically sends connection requests to a list of LinkedIn profiles.
Search Results
LinkedIn search yields millions of results, with a regular account allowing for up to 1000 results in batches, and a scraper can potentially scrape 2500 results individually.
How to Scrape LinkedIn Data?
Scraping LinkedIn data involves the use of automated tools or scripts to extract valuable information like user profiles, job listings, and company details from the LinkedIn platform. This data can serve various purposes, such as lead generation, talent acquisition, and competitor analysis. However, it’s crucial to recognize that LinkedIn strictly prohibits data scraping and has implemented measures to deter users from engaging in it.
The general steps for scraping LinkedIn data are as follows:
- Configure search filters based on specific criteria like job title, location, or company name.
- Employ a LinkedIn scraper tool to crawl public LinkedIn profiles and extract information such as names, job titles, company names, industries, and skills.
- Retrieve the public profile web page and extract the required data.
- Transform the information into a suitable file format, typically CSV, using smart cookies.
- Save the scraped data in a local file or database for future use.
Adhering to best practices is essential when scraping LinkedIn data, which includes respecting rate limits and abstaining from unethical scraping practices. Compliance with LinkedIn’s terms and conditions is of utmost importance, and seeking legal advice in cases of uncertainty is recommended.
Here are some best practices for LinkedIn data scraping to ensure compliance with LinkedIn’s terms of service:
- Avoid scraping data for invading people’s privacy or engaging in malicious activities.
- Use scraping tools committed to ethical standards.
- Thoroughly review and understand the terms of service of any platform before conducting data scraping.
- Show respect for users’ privacy.
In conclusion, LinkedIn data scraping can be a valuable tool for sales, marketing, and recruitment teams, but strict adherence to best practices and LinkedIn’s terms and conditions is necessary to prevent legal or ethical complications.
How Does Artificial Intelligence Help in Efficient Scraping LinkedIn Data?
The application of AI (Artificial Intelligence) can improve the accuracy and efficiency of automated LinkedIn scraping. AI can be used in a variety of ways during the scraping process, including:
Natural Language Processing (NLP)
Natural Language Processing (NLP) is an AI field that uses natural language interaction to extract valuable information from unstructured data sources, improving data accuracy.
Machine Learning (ML)
Machine Learning (ML) is AI that enhances performance by learning from data, such as LinkedIn data, to identify patterns and improve scraping efficiency.
Image Recognition
LinkedIn profile images aid in identifying individuals and improving data accuracy through AI-based face recognition algorithms, linking them to specific profiles.
Sentiment Analysis
Sentiment Analysis uses AI-based algorithms to determine the emotional tone of LinkedIn profiles, providing valuable insights for organizations and recruiters seeking individuals with specific personality traits or views.
Preferred Partner for High Growth Company - Scrape Data Easily Without Coding
Scraping data from websites no longer requires coding expertise. With AI-driven web scraping tools, you can effortlessly extract valuable information from the web. Our AI data scraper offers can easy-to-use interface for all users.
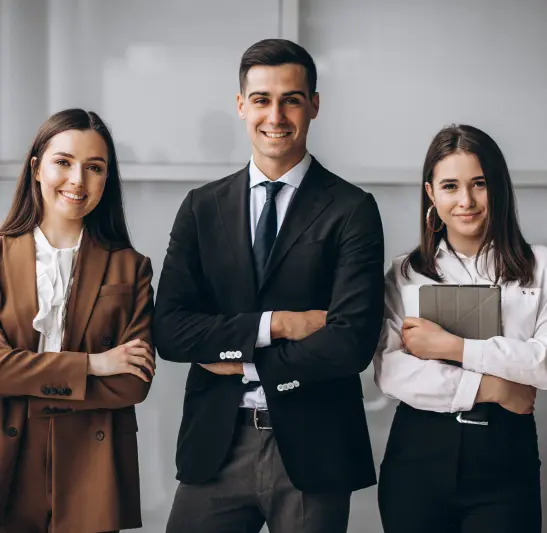
AI-driven Web Scraping
Pre-built Automation
Built-in Data Processing
Quick Deployment
Benefits of Scraping LinkedIn Data
LinkedIn scraping provides up numerous chances for salespeople, growth hackers, and marketers alike:

1.Contact the Right People
A LinkedIn scraping tool can help you identify and collect the contact information of possible clients, business partners, or workers.
3. Determine Your Profile Viewers
You can use a LinkedIn scraper to determine who is viewing your profile and then contact them accordingly.
5. Create a Database
Using a LinkedIn scraper, you can create a database of possible clients, employees, or business partners.
2. Generate Leads
Scraping LinkedIn data can assist you in generating targeted leads by scraping the contact information of users who fit your target criteria.
4. Investigate Your Competitors
By scraping LinkedIn data, you may investigate your competitors by discovering who their employees are, what talents they possess, and so on.
Digital Transformation and Its Effect on LinkedIn Scraping
Digital transformation has significantly impacted LinkedIn scraping by introducing modern technology and tools for efficient data collection and analysis.
1. Cloud Computing : Cloud computing simplifies LinkedIn scraping by storing and processing large data volumes, making it cost-effective and flexible for organizations to manage their data efficiently.
2. Big Data Analytics : Digital transformation has led to a surge in data generation from sources like LinkedIn, enabling big data analytics technologies to provide valuable insights for improved decision-making and overall performance.
3. Artificial Intelligence (AI) : AI-based tools like machine learning and natural language processing can enhance LinkedIn scraping accuracy, providing organizations with a competitive advantage by faster and more precise data analysis.
4. APIs and Integrations : LinkedIn’s APIs enable third-party apps to access its data, simplifying integration into existing systems, automating procedures, and boosting productivity.

LinkedIn Data Scrapers Can Face Some Challenges
Scraping LinkedIn data is a difficult operation, like web scraping. Hence, it is critical that you understand the techniques LinkedIn employs to prevent unauthorized scraping. You must scrape cautiously while respecting the company’s or country’s limits and not scrape any personal data, intellectual property, or confidential information.
LinkedIn opposes scraping when done without authorization (unauthorized access). It puts public-facing websites at risk, such as e-commerce, news sites, and social media sites, and you have no way of tracking where the data travels or how it is used. Unauthorized scraping is not a breach of security or hacking. However, when scraped data falls into the hands of a bad actor, it may be used in unexpected ways.
LinkedIn employs artificial intelligence and legal approaches to prevent unauthorized access and punish violators accountably. These are the obstacles that the web scraper faces when gathering information from websites.

1. Scraping LinkedIn Data Without Logging In
LinkedIn uses automated profile viewing and machine learning models to detect and adapt to new assault patterns, continuously retraining and deploying these models daily.
2. Scraping LinkedIn Data with Log In
LinkedIn uses deep learning and outlier detection to monitor bot-like activities and prevent logged-in scraping, while also implementing additional defenses to detect and remove phoney accounts.
3. Rate Limit Constraint
LinkedIn has a rate limit on automation, requiring authorization and authentication for all applications to collect or scrape member data. This ensures service stability and prevents misuse. There are two types of rate limits:
- Application : the total number of API or scraper calls that can be made in a day.
- Member : the maximum number of calls per day that a member can make per application.
LinkedIn aims to connect genuine individuals with real information, rewarding users with increased visibility. However, new connection requests are restricted for accounts with no connections, education, work experience, or profile photo.
Use Cases of Scraping LinkedIn Data
LinkedIn data points enable a more targeted and efficient approach to business. The following use cases make use of the data you scrape.
1. Reach Out to Right People: LinkedIn is a valuable platform for identifying potential consumers and creating leads through automated scraping queries, filtering profiles based on company names, shared relationships, or resume information.
2. Scrape Data to Find Talents : Human resource experts use scrapers to gather data on employee titles and profiles from specific industries, such as Chief Information Officers with over 10 years of gaming industry expertise.
3. Identify New Deals : LinkedIn data is used by venture capitalists, financial hedge funds, and financial groups to identify underperforming companies, assess their growth potential, and finalize investment decisions.
4. Explore Location Based Markets : LinkedIn public data aids multinational corporations in identifying new markets, launching products, and transforming business practices by gathering data on shared marketing campaigns and influencers, converting it into practical insights.
Trends in LinkedIn Scraping
1. Emphasis on Data Quality : As data privacy requirements become more stringent, there is an increasing emphasis on gathering accurate and high-quality data. To assure data accuracy, LinkedIn scraping solutions are now including capabilities such as data validation and verification.
2.Use of AI and Machine Learning : To automate data collection and analysis, advanced LinkedIn scraping applications incorporate AI and machine learning algorithms. This helps to eliminate human mistakes and boost data processing efficiency.
3. Integration with Other Tools : LinkedIn scraping tools are becoming more integrated with other products and platforms, such as CRM, ATS, and email marketing software. Businesses can use this to streamline their lead generating and sales operations.
4. Compliance with Data Privacy Laws : With the adoption of data privacy rules such as GDPR and CCPA, there is an increasing understanding of the need for data privacy compliance. To comply with these requirements, LinkedIn scraping programs are including features such as data consent management and data erasure.
5. Social Selling and Account-Based Marketing : As businesses seek to target specific individuals or accounts, LinkedIn scraping tools are increasingly being used for social selling and account-based marketing. Advanced LinkedIn scraping techniques can aid in the identification of important decision-makers and influencers inside specific accounts.

Our Technology Partners
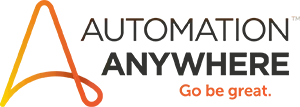
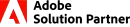
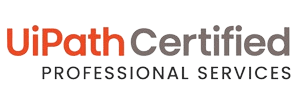
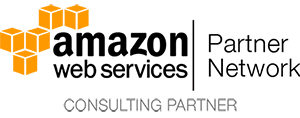
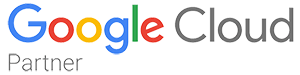
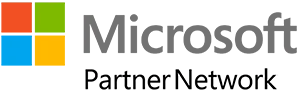
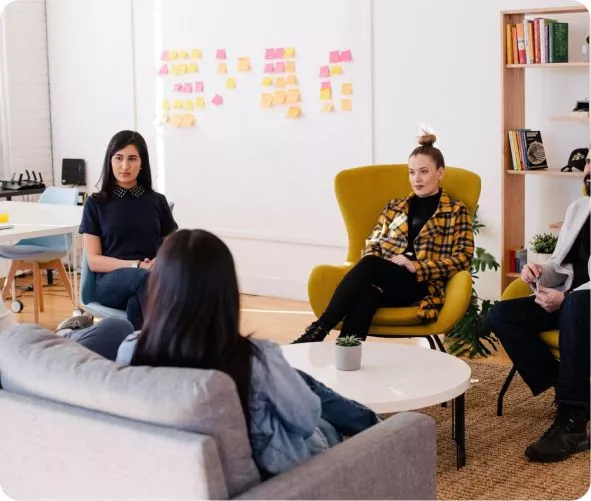
Preferred Partner for High Growth Company
Our 12+ years of experience in price scraping and adaption of the latest algorithms such as Artificial Intelligence, Machine Learning and deep learning for catering the needs of retailers makes us the preferred partner for a high growth company.
%
Customer satisfaction
Years Of Experience
Projects Delivered
Happy Clients & Growing
Quality, Security and Privacy Compliance
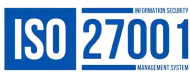
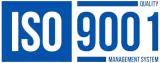
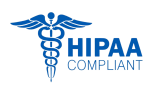
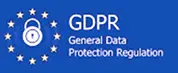
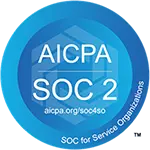