10x Faster With AI
Data Validation services
10x Faster With AI
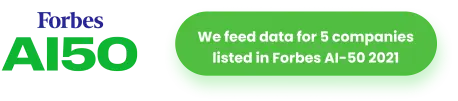
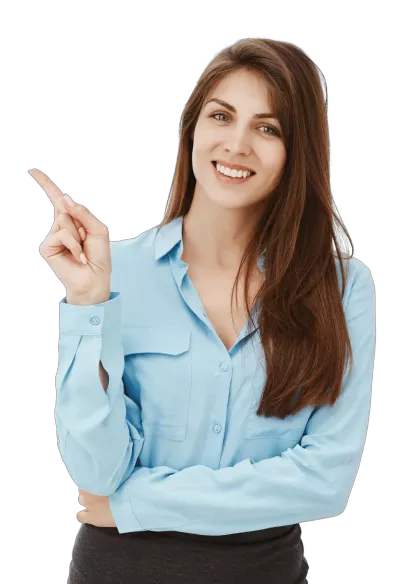
AI-Driven Data Extraction
Real-time Data Updates
Seamless Data Integration
High-Level Accuracy
Anti-Blocking Mechanisms
Customizable Extraction Rules
NO SET-UP COST
NO INFRA COST
NO CODING
4X
Rapid increase of your wealth
30%
Decrease your expenses wisely
1M+
Trusted regular active users
USED BY
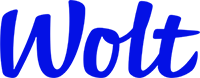
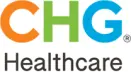


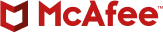

Outsource Bigdata’s AI-driven Data Validation Services to Ensure Accuracy and Reliability
In today’s data-driven era, accurate and reliable information plays a crucial role in making business decisions. Any business or organization’s success depends on the type of data it relies on. There is a continuous rise in data volumes and so the task of upholding the integrity and authenticity of this data has become a real challenge. This is where data validation services come in.
At Outsource BigData, we recognize that data validation is an important part of every organization, whether it be customer information, financial data, or market insights. Our AI-powered data validation services offer a smooth and dependable data validation process, saving you time and money while enhancing data quality.
Our professional team can swiftly find and correct errors, inconsistencies, and duplication in your datasets using sophisticated AI tools. We ensure that your data is compliant with industry standards and regulatory requirements, allowing you to rely on data-driven insights with confidence.
Join us on this data excellence journey, where AI technology fuels your data validation needs, giving actionable and trustworthy information for enhanced decision-making. Outsource Bigdata’s AI-driven data validation services provide assurance of data accuracy, allowing you to unlock the entire potential of your data.
What is Data Validation?
The process of evaluating data for quality, consistency, and integrity is known as data validation. It entails running numerous checks, validations, and tests on the data in order to detect mistakes, anomalies, and discrepancies. Data validation ensures that data fulfills set criteria and requirements.
Process of AI-Driven Data Validation

Determine Data Sampling
In the event of a copious data reservoir necessitating validation, it is imperative to acquire a specimen, rather than subjecting the entire dataset to scrutiny. To optimize the likelihood of project triumph, it is imperative to gain an intricate comprehension of the data sample’s magnitude and concurrently appraise the margin of fallibility.
Validate Database
During the process of Database Authentication, it is of paramount importance to affirm that the extant database meets all prerequisites. To facilitate this confirmation, it is imperative to determine congruence between the source and target data fields, ascertain the uniqueness of identification codes, and enumerate the quantum of records.
Validate Data Format
The essence of data adequacy and the variability requisite for the target validation must be delineated in the preliminary phase, followed by a meticulous pursuit for irregularities, duplication of data, erroneous data structures, and the manifestation of vacant field values.
Types of Data Validation
Validating data properties like type, range, format, and consistency requires code or specialized tools, with some tests executed depending on the application and data.

Type Check
This technique ensures data input matches the correct data type, rejecting any data with letters or special symbols deviating from this standard.
Format Check
Format check, on the other hand, scrutinizes if the entered data complies with a predefined format. For instance, a date column may be expected to adhere to a fixed format like “YYYY-MM-DD” or “DD-MM-YYYY.”
Consistency Check
Consistency check verifies that data entries are logically consistent. It is exemplified in scenarios like confirming that a delivery date occurs after the shipping date for a parcel.
Range Check
A range check is used to verify if input data, like latitude and longitude, falls within a specific range, excluding values outside of this range
Code Check
The Code check method scrutinizes whether the entered data adheres to predefined codes. For instance, a field may exclusively accept a predetermined set of codes associated with a particular product.
Uniqueness Check
The uniqueness check ensures the uniqueness of specific data entries, such as employee IDs or bank account numbers, to prevent operational complications during data processing and management.
Preferred Partner for High Growth Company - Scrape Data Easily Without Coding
Scraping data from websites no longer requires coding expertise. With AI-driven web scraping tools, you can effortlessly extract valuable information from the web. Our AI data scraper offers can easy-to-use interface for all users.
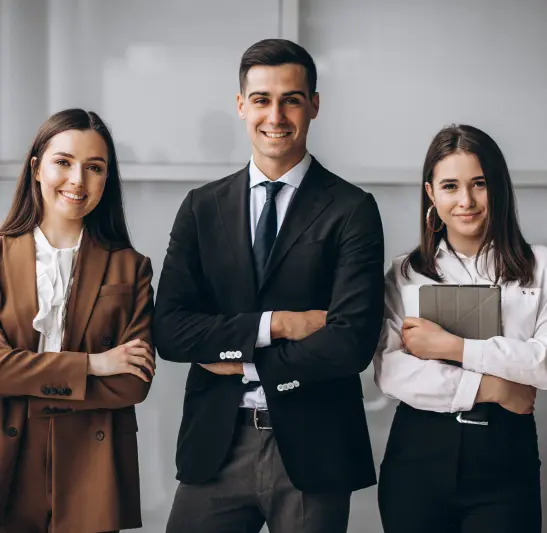
Methods of Data Validation
There are several data validation methods available, and each approach contains unique elements for the best data validation process. These methods are as below:
Script Validation
The validation procedure is carried out using a programming language such as Python, which is utilized to write the whole validation script. You can compare data values and structure to your set rules to guarantee that all relevant information is within the appropriate quality parameters. Depending on the complexity and amount of the data collection being validated, this type of data validation might be time-consuming.
Program Validation
There are numerous software programs available to assist you in validating data. This kind of validation is quite straightforward because these programs have been designed to grasp your rules and the file formats you are working with. The perfect tool will enable you to include validation at every stage of your workflow without having a comprehensive understanding of the underlying format.
The following programs are available for use:
A) Open-Source Software
In the contemporary landscape, a myriad of enterprise tools have emerged, catering to the validation and rectification of data. These solutions, while esteemed for their security and stability, do entail a more substantial financial investment and necessitate additional infrastructure to function optimally.
B) Enterprise Tools
An alternative approach entails leveraging open-source tools. This avenue is cost-effective, primarily because many of these tools operate within the cloud. However, it is imperative to note that proficiency is required to harness them effectively, making knowledge a prerequisite for their successful utilization.
Benefits of AI-Driven Data Validation Services
1. Enhanced Accuracy: AI algorithms excel in swiftly and accurately analyzing substantial data volumes, thereby mitigating the risk of human errors in the process.
2. Enhanced Efficiency: AI-powered data validation automates manual validation, saving time and effort, enabling reallocation of human resources towards creative and strategic business pursuits.
3. Cost Savings: Automated data validation reduces costs by eliminating the need for human validators and outsourcing services to reputable providers for efficient and expeditious validation.
4. Improved Data Throughput: AI-driven data validation systems demonstrate the capacity to handle extensive and intricate datasets, facilitating the rapid and seamless validation of copious data.
5. Real-time Vigilance: AI-powered data validation monitors real-time data, detecting defects and delivering reliable software at an accelerated pace.
6. Improved Productivity: It enhances data quality, enabling businesses to make informed decisions, while selecting the right partner optimizes time and cost efficiency, resulting in a higher return on investment.

Challenges Faced by Data Validation Services

Dealing with Large & Varied Datasets
Validating data from various sources, formats, and domains, including structured, unstructured, and semi-structured data, can be a time-consuming, resource-intensive, and error-prone task.
Handling Missing & Noisy Data
Incomplete or erroneous data have critical impact on data validation, necessitating meticulous handling and appropriate techniques for addressing missing entries or errors.
Ensuring Data Security & Privacy
AI and machine learning applications require data protection from unauthorized access, tampering, and disclosure while respecting the rights and preferences of data owners or subjects.
Monitoring Data Drift & Bias
Data is dynamic, requiring constant monitoring and correction for changes in distribution or biases that could compromise model and algorithm integrity
Lack of Control over Data Collection & Implementation
Due to the team’s lack of direct involvement in data collection and implementation, resolving issues at their source becomes difficult.
Understanding & Rectifying Root Issues
Identifying and rectifying data non-compliance with validation criteria is a complex and time-consuming task, especially in an increasingly complex data ecosystem.
Trends in Data Validation
- Digital Transformation Dynamics: Digital technologies are transforming data validation, integrating data analytics, aiming to improve efficiency, cost-effectiveness, and risk management in quality assurance.
- Elevated Significance of Data Quality Management: In 2023, ‘Data Quality’ will gain prominence, distinguishing it from ‘Data Integrity’, which focuses on information reliability and validity.
- Agility and Swift Value Realization: Modern business leaders prioritize agility and value realization, requiring precise, uniform, and contextually relevant information, with data integrity and validation being key pillars.
- Enlarged Data Integrity Competencies: Enterprises are actively enhancing their data integrity competencies, acknowledging the persistent challenges in maintaining high levels of data integrity.
- Challenges in Data Volume, Variety, and Velocity: Cloud computing offers scalability and elasticity, but it also increases data volumes, exacerbates the complexities of data validation due to the growing scale and diversity of data streams.
- Data Validation for Regulatory Compliance: Data validation is crucial for maintaining regulatory compliance in industries like healthcare and finance, ensuring accuracy, efficiency, and risk mitigation, making it a cornerstone of operational frameworks.

Our Technology Partners
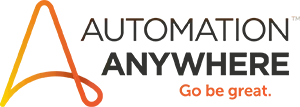
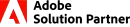
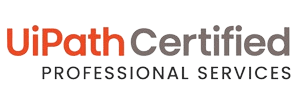
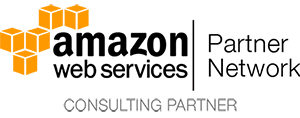
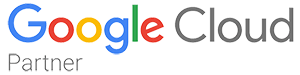
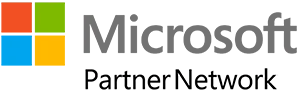
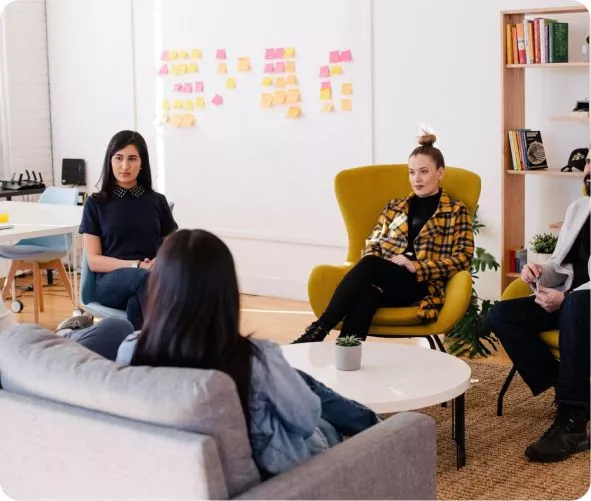
Preferred Partner for High Growth Company
Our 12+ years of experience in price scraping and adaption of the latest algorithms such as Artificial Intelligence, Machine Learning and deep learning for catering the needs of retailers makes us the preferred partner for a high growth company.
%
Customer satisfaction
Years Of Experience
Projects Delivered
Happy Clients & Growing
Quality, Security and Privacy Compliance
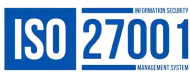
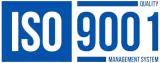
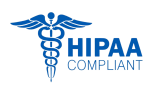
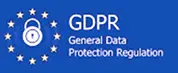