Data Management Services
10x Faster
With AI
10x Faster With AI
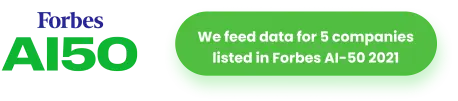
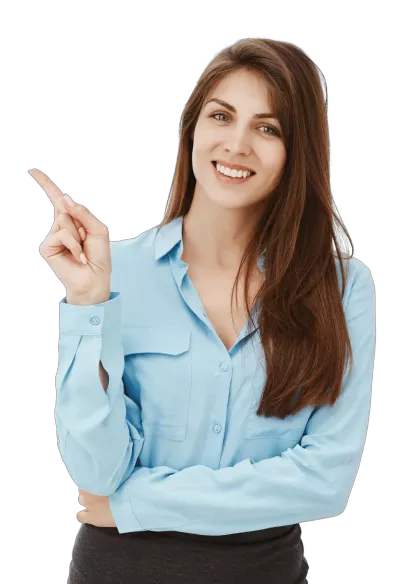
AI-Driven Data Extraction
Real-time Data Updates
Seamless Data Integration
High-Level Accuracy
Anti-Blocking Mechanisms
Customizable Extraction Rules
NO SET-UP COST
NO INFRA COST
NO CODING
4X
Rapid increase of your wealth
30%
Decrease your expenses wisely
1M+
Trusted regular active users
USED BY
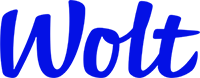
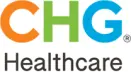


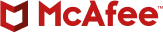

Efficiently Manage Your Data with Our Expert Data Management Services
AI has the potential to revolutionize nearly every aspect of life, including how people work, study, travel, govern, and engage in recreational activities. However, in order to fully benefit from everything AI has to offer, enterprises must embed AI at the data level. Additionally, they must ensure that AI supports the entire data management lifecycle. This includes ingestion to curation and discovery, as well as driving applications built on that data.
Managing data is no longer a daunting task with AI-Augmented data management services. Outsource Big Data’s team has over 10 years of experience providing businesses all over the world with end-to-end data management solutions. We leverage the latest technologies to help you stay ahead of the curve and get the most out of your data.
Streamline your organization’s operations with our extensive suite of features and get an edge over your competition. Get ready for the future of data automation with our groundbreaking outsource data management services that make it easy for you to stay ahead of the curve!
What is Data Management?
Data management is the professional practice of establishing and maintaining a framework for the ingest, storage, retrieval, and preservation of data that is critical to modern business operations. It is the core component that connects all stages of the information lifecycle.
Outsource BigData is one of the best data management services that helps to speed up business growth with the help of automation.
Why is Data Management Important?
Data management is a first important step towards implementing effective data analysis at scale. This leads to critical insights that add value to your customers and improve your bottom line. People across an organization can find and access trusted data for their queries with effective data management. Some of the advantages of good data management services are as follows:

Enhances Reliability and Security
Because of regulations, employees can be confident that data is always available and of high quality. Companies that have reliable, up-to-date data can more effectively adapt to market changes and client demands.
Outsource data management services reduce potential errors by defining processes and policies for usage. Also, they build trust in the data used to make decisions throughout your organization. Moreover, companies with reliable, up-to-date data can respond to market changes and customer needs more efficiently.
Understand Your Potential Customers
Who are the most profitable customers for your business? This answer may not be as simple as it sounds for a modern technology company. You cannot simply examine which customers spend the most money with you.
You must also consider the cost of supporting those customers. This information could come from your help desk software or your payroll system. Furthermore, larger customers are likely to be more expensive to acquire. Your CRM, marketing automation, and advertising platforms enable deriving information. Data management services help you to get all this information easily by accurately sorting and organizing the data.
Assessment of Customer Acquisition Channels
When it comes to acquisition costs, it’s critical to understand which channels need reinvestment.
A data warehouse in data management companies enables you to link customer acquisition costs to customer retention data. This allows you to see your total ROI at a glance.
Enhances Transparency in Business Processes
Companies collect so much data that it’s difficult to make sense of it all. Combining this data with complex business processes, lead to a lack of trust.
However, clear data management allows employees and clients to get immediate answers. This transparency has the potential to increase engagement, boost innovation, and improve communication. Data management services help to improve transparency in business processes.
Helps to Scale Your Business
Data management services enable organizations to effectively scale data and usage occasions through repeatable processes that maintain data and metadata. When processes are simple to replicate, your company can avoid the unnecessary costs of duplication. It includes employees conducting the same research over and over or re-running costly queries.
What are Types of Data Management?
In an organization’s data environment, data management plays various roles, simplifying crucial functions and saving time. Here are the types of data management:
Data Pipeline
A data pipeline is a crucial process in modern data-driven organizations, involving the collection and transfer of raw data from various sources for analysis.
Data Modelling
Data modeling is the act of crafting a visual representation of an entire information system or its components to convey the relationships between data points and structures.
Data Architecture
A data management architecture is a set of models, policies, rules, and standards used by an organization to manage and control data storage, utilization, and security.
Data Integration
Data management services enable businesses to integrate real-time data through methods like data capture changes, streaming integration, and data virtualization, reducing physical data loading.
Data Governance
A data governance framework governs an enterprise’s information state, establishing policies for information intake, flow, and protection. Data governors manage stewards, quality management professionals, and security teams, supporting a master data management approach.
Data Lifecycle Management (DLM)
DLM or Data Lifecycle Management helps you get the most out of your data until it’s deleted. It is defining, organizing, and creating policies for data management at every stage of its life.
Below are the stages of DLM:
- Collection
- Access
- Usage
- Storage
- Transfer
- Deletion or Destruction
Data Storage
Data storage before or after processing depends on data type and purpose, necessitating a defined analytics schema and collaboration between business users and data engineers.
Data Catalogues
Data catalogues are inventories of a company’s data resources, organized using metadata, aiding data professionals in quickly locating suitable data for various analytical or business objectives.
Data Migration
Data migration involves transferring data from one storage system to another, often used for replacing servers, consolidating or decommissioning a data center, or for other purposes.
Data Processing
Raw data ingestion occurs from various sources, such as web APIs, mobile apps, IoT devices, forms, and surveys. It is then processed using ETL or ELT techniques.
Data Security
Modern businesses are implementing security measures to protect customer data from cybercriminals and disaster recovery incidents, eliminating unauthorized access, corruption, and theft of digital information.
ETLs (Extract, Transform, Load)
ETLs or Extract, Transform, Load are a popular data pipeline type. It the procedure of collection data from various sources into a centralized repository known as a data warehouse. There are three stages during the process:
Extraction: It is the extraction of the relevant data from the source database.
Transformation: It is modifying the data to make it more suitable for analytics.
Loading: It is transferring the data into the target database.
Preferred Partner for High Growth Company - Scrape Data Easily Without Coding
Scraping data from websites no longer requires coding expertise. With AI-driven web scraping tools, you can effortlessly extract valuable information from the web. Our AI data scraper offers can easy-to-use interface for all users.
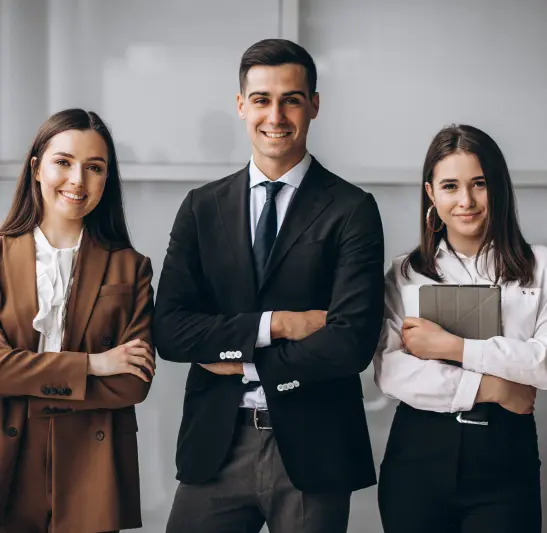
Limitations of Data Management

1. Lack of Data Insight
Without proper implementation, data collection is meaningless. Mining data includes a variety of sources such as smart devices, sensors, video cameras, social media, and so on.
4. Changing Data Requirements
Data compliance requirements are extremely complex and constantly changing. It is important to continuously review data to keep data up to date with new regulations. Regular monitoring of personally identifiable information (PII) is essential for compliance.
2. Effective Storage of Data
The format of data storage depends on that required in data lakes or data warehouses. Data scientists must be able to work under time constraints in order to transform data into the required format for storage. Data structure is possible into a variety of shapes and models. Only proper formatting can make the data usable for analysis.
5. Processing and Converting Data
To properly utilize the collected data, data processing is crucial. If data processing and conversion take a long time, the data becomes obsolete and proper data analysis is not possible. This means that the data gathered will be useless to the organization.
3. Performance Level Maintenance
More data collection means a more extensive database. An organization should strive to maintain a peak response time by changing indexes on a regular basis and monitoring the queries answered by the database. This may make it difficult to maintain the organization’s performance level
6. Optimizing IT Agility and Costs
Many online options for previously offline systems have emerged with the advent of modernization in the business sector. Data storage is now possible in the cloud, on-premises, or in a hybrid mode.
Role of a Data Engineer in Data Management
Data engineers manage and organize data, identifying trends and inconsistencies to impact business goals. They require technical knowledge in programming, mathematics, and computer science, as well as soft skills to communicate trends and assist in data utilization.
- Designing, building, testing, and maintaining architecture is a must.
- Align the architecture with the needs of the business.
- Data collection
- Create data set processes.
- Make use of programming languages and tools.
- Determine methods to improve data dependability, efficiency, and quality.
- Conduct research to find answers to industry and business questions.
- Use large data sets to solve business problems.
- Use advanced analytics software, machine learning, and statistical methods.
- Data preparation for predictive and prescriptive modelling.
- Using data, discover hidden patterns.
- Utilize data to identify tasks for automation.
- Provide analytics-based updates to stakeholders.

Data Fabric - The Solution to Data Management Challenges
Companies are stockpiling data in silos, often unusable. Gartner predicts data fabric technologies will be four times more efficient by 2024, reducing human-driven data tasks and integrating data silos, addressing data governance issues.
A data fabric is a long-term, scalable solution for managing data collection, governance, integration, and sharing, helping organizations become digital leaders and address challenges.
Implementing a data fabric can assist a company in meeting its data management challenges as below:
- Using a single interface to access and collect all data, no matter where it is located.
- Providing healthier, more trustworthy data faster by eliminating many tools. As a result, data integration, quality, governance, and sharing become simpler and unified.
- Increased scalability to accommodate growing data volumes, data sources, and applications.
- Supporting on-premises, hybrid, and multi-cloud environments, as well as providing faster migration between them.
- Relying less on legacy infrastructures and solutions.
- Data management infrastructure can be future-proofed by adding new sources and ends. Furthermore, adding new technologies to the data fabric without disrupting existing deployments or connections.
Future Of Data Management
New technologies such as the cloud, NoSQL, and knowledge graphs are reshaping data analytics and development paradigms. Tools with machine learning and artificial intelligence capabilities have revolutionised data management workflow automation.
1. Increasing Regulatory Requirements: It’s worth noting that the maximum fine for a GDPR violation can reach 4% of annual global turnover, so there’s theoretically no upper limit.
2. Data Governance: It is not possible to leave data governance to the well-intentioned but overburdened data steward. It is important to instil domain-first principles and standards throughout the data team.
3. Data Mesh: One goal of the data mesh is to empower individual teams by creating a self-service data platform where they can own information about their area of business.
4. Data Transformation or ELT: Extraction and transformation no longer require a single line of code thanks to automated data pipeline and transformation tools.
5. Data Streaming / Transportation: Streaming is now a fundamental component of how to create new modern applications. This has significant implications for the future of data management.
6. AI Model: Artificial intelligence can now detect and eliminate incomplete, duplicate, or irrelevant data stored across multiple clouds. You don’t have to be a data scientist to use AI to get the most out of your data.

Our Technology Partners
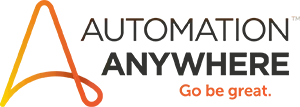
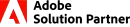
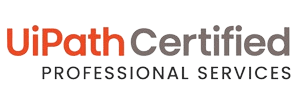
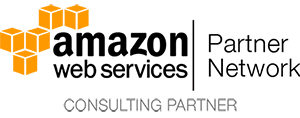
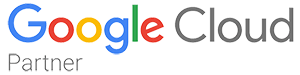
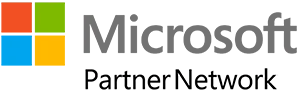
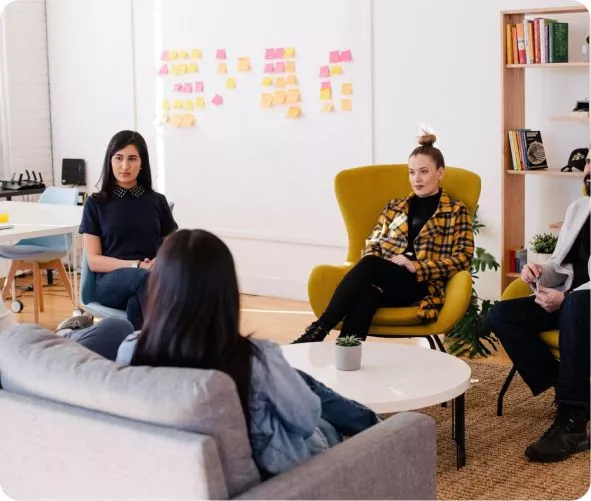
Preferred Partner for High Growth Company
Our 12+ years of experience in price scraping and adaption of the latest algorithms such as Artificial Intelligence, Machine Learning and deep learning for catering the needs of retailers makes us the preferred partner for a high growth company.
%
Customer satisfaction
Years Of Experience
Projects Delivered
Happy Clients & Growing
Quality, Security and Privacy Compliance
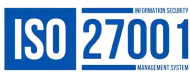
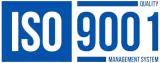
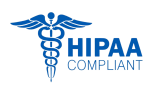
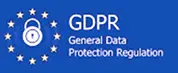